Unlocking Efficiency with Data Labeling Tools: A Comprehensive Guide
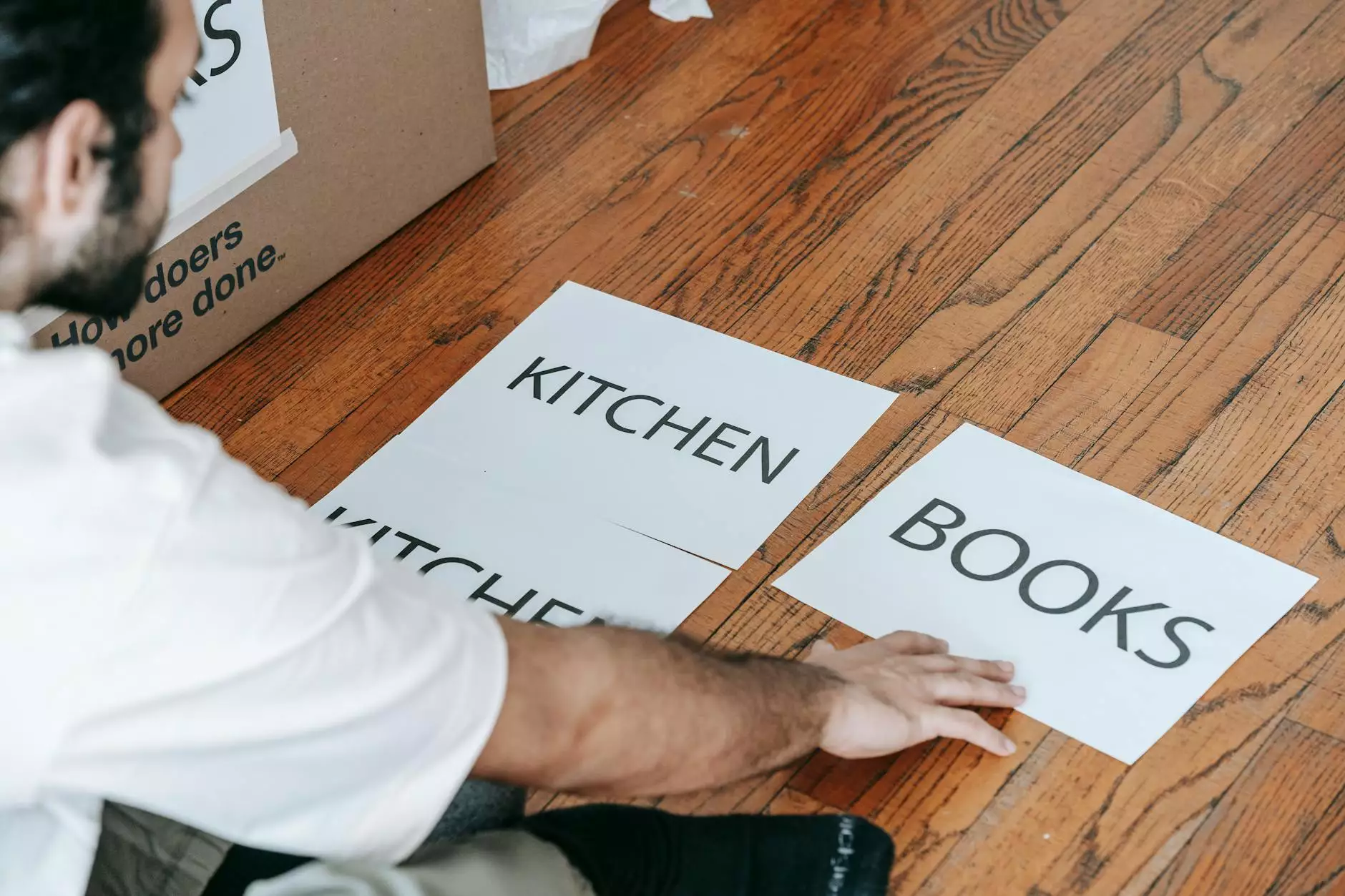
In the rapidly evolving landscape of technology, the significance of data labeling tools has surged to new heights. As businesses increasingly rely on data-driven strategies, the need for precise and efficient data labeling has become paramount. This article delves into the intricacies of data labeling, its pivotal role in software development, and how tools can transform the approach to data management. Let’s explore the multifaceted world of data labeling and its impact on your business.
Understanding Data Labeling
Data labeling refers to the process of assigning meaningful tags or labels to raw data, making it easier for machine learning algorithms to interpret and learn from it. This process is crucial for various applications, including:
- Natural Language Processing (NLP)
- Image and Video Analysis
- Speech Recognition
- Sentiment Analysis
Without accurate data labeling, machine learning models may struggle to recognize patterns, leading to subpar performance. Thus, businesses must invest in a reliable data labeling tool to ensure their data is well-organized and interpretable.
The Importance of Data Labeling in Software Development
For companies like Keymakr, which fall under the umbrella of software development, the efficacy of software products relies heavily on the quality of data processed through these systems. Here’s why data labeling is at the core of software development:
1. Enhancing Machine Learning Models
One of the primary applications of data labeling is in training machine learning models. Labeling data accurately allows algorithms to learn from examples and make informed predictions. This results in:
- Increased accuracy of the model's predictions
- Improved reliability in real-world applications
- Faster development cycles due to iteration on labeled datasets
2. Streamlining Data Processing
Efficiently organized data facilitates easier access and management. A robust data labeling tool can streamline the processing of vast amounts of data, allowing businesses to:
- Quickly retrieve and utilize data for analyses
- Improve collaboration between teams through shared labeled datasets
- Save time and resources in data preparation for development projects
Features to Look for in a Data Labeling Tool
Choosing the right data labeling tool can make or break your data management process. Here are key features that should be considered:
1. User-Friendly Interface
A tool with an intuitive interface increases user adoption and reduces training time. Look for options that are easy to navigate, allowing team members to focus on labeling rather than figuring out how to use the tool.
2. Customization Options
The ability to tailor the tool to fit specific business needs is invaluable. Customizable workflows, labeling formats, and templates can significantly enhance productivity.
3. Scalability
A good labeling tool should easily scale with your data needs. As your projects grow, your tool should accommodate increased data volume without compromising performance.
4. Integration Capabilities
Look for tools that integrate seamlessly with your existing software and infrastructure. Strong integrations enhance workflows, allowing for efficient data transfer and management.
5. Collaboration Features
In software development, collaboration is critical. Opt for data labeling tools that enable multiple users to work together, providing features such as commenting, version control, and task assignment.
Best Practices for Effective Data Labeling
To maximize the benefits of your data labeling tool, consider implementing the following best practices:
1. Define Clear Guidelines
Establishing clear labeling guidelines ensures consistency across datasets. This clarity helps minimize errors and establishes a uniform standard for all data contributors.
2. Utilize Active Learning
Incorporate active learning methodologies where models suggest labels which humans then verify. This process not only enhances the labeling efficiency but also improves annotation quality over time.
3. Regularly Audit Labeled Data
Conduct audits on labeled data regularly to ensure ongoing quality. Implement feedback loops to address common issues and refine your data labeling processes.
4. Invest in Training
Providing training for team members involved in data labeling can significantly enhance the accuracy and consistency of data. Continuous skill development should be prioritized.
Conclusion: The Future of Data Labeling Tools
The future of data labeling tools is bright, as advancements in artificial intelligence and machine learning continue to emerge. Businesses that invest in high-quality data labeling are likely to see a substantial return on investment as they harness the full power of their data. By choosing the right tools, adopting best practices, and prioritizing data management, companies can drive significant progress and innovation in software development. As industries become more data-centric, ensuring effective and efficient data labeling will remain a key component for competitive advantage.
For businesses like Keymakr, embracing robust data labeling tools means setting the stage for success in an increasingly data-driven world. It’s time to leverage labeling solutions to unlock the full potential of your data and elevate your software development process.